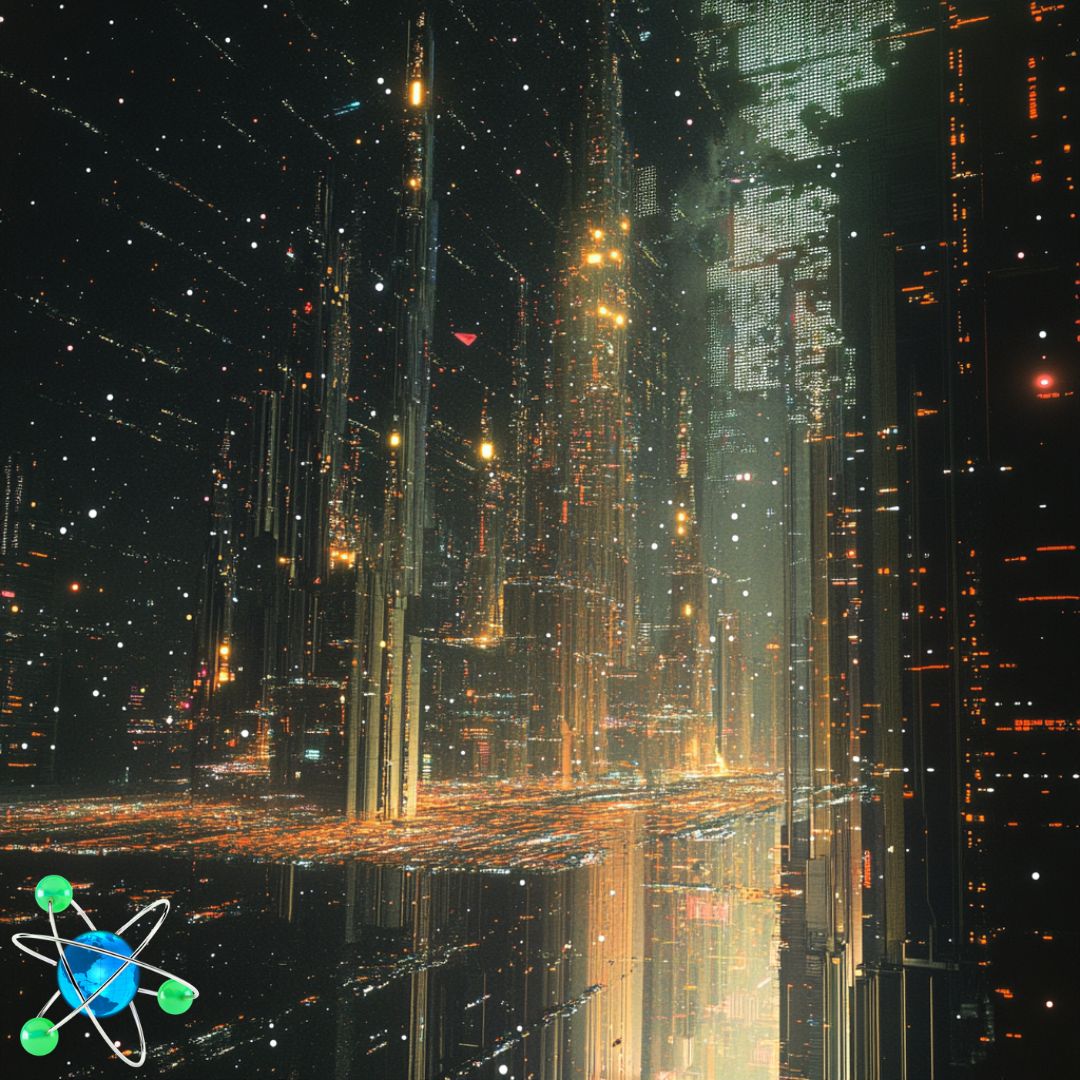
-
Data Exhaustion in AI: Elon Musk claims that AI companies have exhausted the pool of human-generated data needed to train advanced models, signaling a shift toward synthetic data created by AI itself.
-
Risks of Synthetic Data: While synthetic data offers a solution, it raises concerns about the quality and reliability of AI outputs, with issues like “hallucinations” and the potential for model collapse due to over-reliance on AI-generated content.
-
Legal and Ethical Implications: The use of synthetic data and copyrighted material in AI training sparks debates over intellectual property rights, with creators demanding compensation for their contributions to model development.
Elon Musk, founder of xAI and one of the most prominent voices in the AI industry, recently suggested that artificial intelligence companies have reached a significant milestone—one that raises pressing questions about the future of machine learning. Speaking in a livestreamed interview on his platform, X, Musk declared that the collective pool of human knowledge available for training AI models has effectively been “exhausted.” According to Musk, this turning point was reached in the past year, and it leaves the field at a crossroads: the only viable way forward may be to rely increasingly on synthetic data, a solution that is as promising as it is fraught with challenges.
AI systems, like the GPT-4 model that powers ChatGPT, are built by training on vast datasets drawn from the internet. These models learn to identify patterns in the data, enabling them to generate human-like text, images, and other outputs. However, the finite nature of high-quality, publicly available information has created a bottleneck. Musk explained that as the reservoir of human-generated content runs dry, synthetic data—material created by AI itself—will become indispensable for training and refining new systems. Already, companies like Meta, Microsoft, OpenAI, and Google have begun incorporating synthetic data into their AI development pipelines. While this strategy is a pragmatic response to data scarcity, it introduces a host of technical, ethical, and practical concerns.
One of the most critical challenges is the risk of “hallucinations,” where AI models generate content that is inaccurate or nonsensical. Musk acknowledged that synthetic data can exacerbate this issue, as it requires AI to assess its own output—a process inherently prone to reinforcing errors or biases. He pointed out the difficulty of ensuring that synthetic content reflects reality, asking, “How do you know if it … hallucinated the answer or it’s a real answer?” This concern underscores a broader issue: when synthetic data forms the foundation of training, the quality of AI outputs may deteriorate over time in a phenomenon researchers refer to as “model collapse.” Andrew Duncan, director of foundational AI at the UK’s Alan Turing Institute, warned that over-reliance on synthetic inputs could lead to diminishing returns, producing biased and uninspired models.
Adding to the complexity is the growing presence of AI-generated content on the internet itself. As these materials circulate, they risk being incorporated into future training datasets, further muddying the line between human knowledge and machine-generated material. This feedback loop could erode the integrity of both AI outputs and the internet as a whole, raising alarm about the long-term consequences of unchecked reliance on synthetic data.
The issue also touches on significant legal and ethical questions. High-quality data has become a prized commodity in the AI arms race, sparking disputes over intellectual property rights. OpenAI has admitted that tools like ChatGPT would not be possible without leveraging copyrighted material. Meanwhile, creators, publishers, and creative industries are demanding compensation for the use of their works in AI training. These tensions highlight a critical question: how can AI companies balance innovation with respect for intellectual property and the need for transparency?
While the challenges are daunting, solutions are beginning to take shape. Some experts advocate for the careful curation of training datasets to ensure their accuracy and relevance, whether they are human-generated or synthetic. Others propose hybrid approaches that combine synthetic data with verified, high-quality human contributions. There is also a push for greater transparency in how AI models are trained, including documenting the origins and nature of the data used.
The road ahead for AI development is both exciting and uncertain. As the field grapples with data limitations, the choices made today will shape not only the capabilities of future AI systems but also their impact on society, creativity, and knowledge itself. Musk’s remarks serve as a stark reminder that the rapid pace of AI innovation demands equally swift attention to its challenges, lest the technology outpace humanity’s ability to guide it responsibly.